RapMixTox: Rapid and automated prediction of complex mixture toxicity by nontarget instrumental analysis
Anneli Kruve, Magnus Breitholtz, Jonathan Martin, Matthew MacLeod
FORMAS 2021 – 2023
The Swedish Government recently launched a comprehensive report on the future of chemical risk management accounting for combination effects and assessing chemicals in groups. The major conclusions from the report were that there is a need to improve the assessment and management of mixture risks and increase and develop group-wise assessment and management of chemicals. In more detail, modelling both co-exposures and combined toxicity was identified as key for regulatory progress.
Unfortunately, assessing the effect of the mixtures traditionally requires expensive and slow testing in vivo, and the constituents of the mixture need to be known. Real-world environmental water samples may contain hundreds if not thousands of chemical compounds, which vary strongly depending on the location and proximity to industrial and agricultural activities. Water samples are routinely analysed using liquid chromatography mass spectrometry, which allows separating the components and measuring several characteristics of these compounds. Still, identification of all of the constituents of an environmental water sample is a very challenging task, and all constituents of a sample do not contribute equally to the mixture effect.
In the current project, we will develop a method to predict the mixture toxicity of water samples without the need for identification of each constituent, and without biological testing. Specifically, we will use the information collected while analysing surface water sample with liquid chromatography mass spectrometry directly for predicting the toxic effect of the compounds. This will allow us to evaluate the mixture toxicity rapidly, without identifying the structure of all chemical compounds in the sample. Moreover, it will become possible to pinpoint the compound(s) with the greatest contribution to the mixture effect prior to the identification of the compounds and without the need for time-consuming and expensive animal testing.
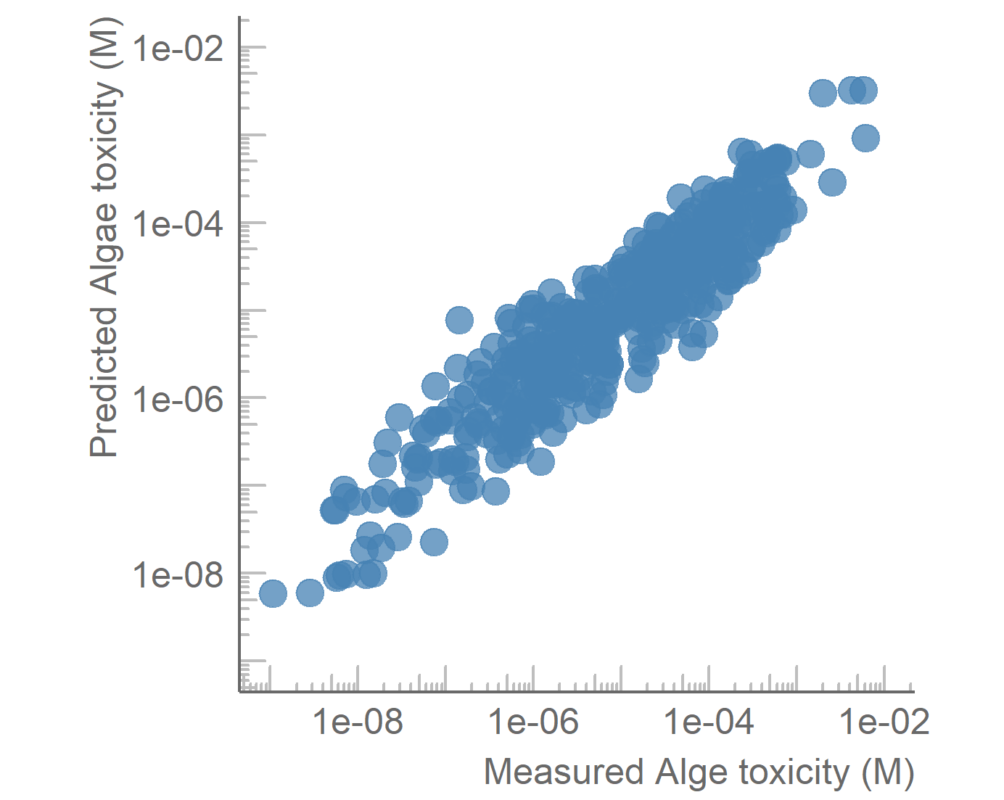