Evaluation of Nontargeted Mass Spectral Data Acquisition Strategies for Water Analysis and Toxicity-Based Feature Prioritization by MS2Tox
Pilleriin Peets, May Britt Rian, Jonathan W. Martin, Anneli Kruve
ES&T 2024
The machine-learning tool MS2Tox can prioritize hazardous nontargeted molecular features in environmental waters, by predicting acute fish lethality of unknown molecules based on their MS2 spectra, prior to structural annotation. It has yet to be investigated how the extent of molecular coverage, MS2 spectra quality, and toxicity prediction confidence depend on sample complexity and MS2 data acquisition strategies. We compared two common nontargeted MS2 acquisition strategies with liquid chromatography high-resolution mass spectrometry for structural annotation accuracy by SIRIUS+CSI:FingerID and MS2Tox toxicity prediction of 191 reference chemicals spiked to LC-MS water, groundwater, surface water, and wastewater. Data-dependent acquisition (DDA) resulted in higher rates (19–62%) of correct structural annotations among reference chemicals in all matrices except wastewaters, compared to data-independent acquisition (DIA, 19–50%). However, DIA resulted in higher MS2 detection rates (59–84% DIA, 37–82% DDA), leading to higher true positive rates for spectral library matching, 40–73% compared to 34–72%. DDA resulted in higher MS2Tox toxicity prediction accuracy than DIA, with root-mean-square errors of 0.62 and 0.71 log-mM, respectively. Given the importance of MS2 spectral quality, we introduce a “CombinedConfidence” score to convey relative confidence in MS2Tox predictions and apply this approach to prioritize potentially ecotoxic nontargeted features in environmental waters.
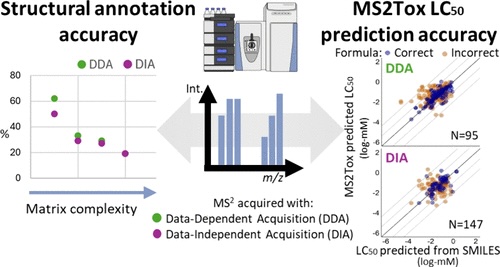
Prioritization, Identification, and Quantification of Emerging Contaminants in Recycled Textiles Using Non-Targeted and Suspect Screening Workflows by LC-ESI-HRMS
Drew Szabo, Stellan Fischer, Aji P Mathew, Anneli Kruve
Anal. Chem. 2024
DOI: 10.1021/acs.analchem.4c02041
Recycled textiles are becoming widely available to consumers as manufacturers adopt circular economy principles to reduce the negative impact of garment production. Still, the quality of the source material directly impacts the final product, where the presence of harmful chemicals is of utmost concern. Here, we develop a risk-based suspect and non-targeted screening workflow for the detection, identification, and prioritization of the chemicals present in consumer-based recycled textile products after manufacture and transport. We apply the workflow to characterize 13 recycled textile products from major retail outlets in Sweden. Samples were extracted and analyzed by liquid chromatography coupled with high-resolution mass spectrometry (LC-HRMS). In positive and negative ionization mode, 20,119 LC-HRMS features were detected and screened against persistent, mobile, and toxic (PMT) as well as other textile-related chemicals. Six substances were matched with PMT substances that are regulated in the European Union (EU) with a Level 2/3 confidence. Forty-three substances were confidently matched with textile-related chemicals reported for use in Sweden. For estimating the relative priority score, aquatic toxicity and concentrations were predicted for 7416 features with tandem mass spectra (MS2) and used to rank the non-targeted features. The top 10 substances were evaluated due to elevated environmental risk linked to the recycling process and potential release at end-of-life.
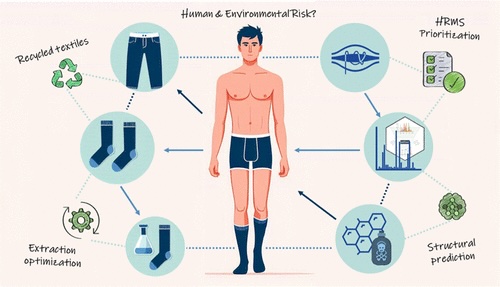
Critical review on in silico methods for structural annotation of chemicals detected with LC/HRMS non-targeted screening
Henrik Hupatz, Ida Rahu, Wei-Chieh Wang, Pilleriin Peets, Emma H Palm, Anneli Kruve
Anal. Banal. Chem. 2024
DOI: 10.1007/s00216-024-05471-x
Non-targeted screening with liquid chromatography coupled to high-resolution mass spectrometry (LC/HRMS) is increasingly leveraging in silico methods, including machine learning, to obtain candidate structures for structural annotation of LC/HRMS features and their further prioritization. Candidate structures are commonly retrieved based on the tandem mass spectral information either from spectral or structural databases; however, the vast majority of the detected LC/HRMS features remain unannotated, constituting what we refer to as a part of the unknown chemical space. Recently, the exploration of this chemical space has become accessible through generative models. Furthermore, the evaluation of the candidate structures benefits from the complementary empirical analytical information such as retention time, collision cross section values, and ionization type. In this critical review, we provide an overview of the current approaches for retrieving and prioritizing candidate structures. These approaches come with their own set of advantages and limitations, as we showcase in the example of structural annotation of ten known and ten unknown LC/HRMS features. We emphasize that these limitations stem from both experimental and computational considerations. Finally, we highlight three key considerations for the future development of in silico methods.
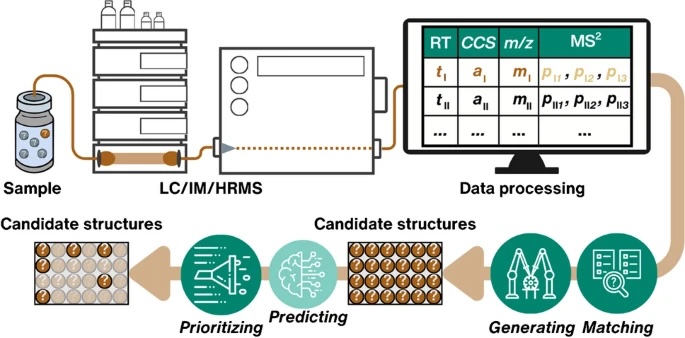
Estimating LoD-s Based on the Ionization Efficiency Values for the Reporting and Harmonization of Amenable Chemical Space in Nontargeted Screening LC/ESI/HRMS
Amina Souihi, Anneli Kruve
Anal. Chem. 2024
DOI: 10.1021/acs.analchem.4c01002
Nontargeted LC/ESI/HRMS aims to detect and identify organic compounds present in the environment without prior knowledge; however, in practice no LC/ESI/HRMS method is capable of detecting all chemicals, and the scope depends on the instrumental conditions. Different experimental conditions, instruments, and methods used for sample preparation and nontargeted LC/ESI/HRMS as well as different workflows for data processing may lead to challenges in communicating the results and sharing data between laboratories as well as reduced reproducibility. One of the reasons is that only a fraction of method performance characteristics can be determined for a nontargeted analysis method due to the lack of prior information and analytical standards of the chemicals present in the sample. The limit of detection (LoD) is one of the most important performance characteristics in target analysis and directly describes the detectability of a chemical. Recently, the identification and quantification in nontargeted LC/ESI/HRMS (e.g., via predicting ionization efficiency, risk scores, and retention times) have significantly improved due to employing machine learning. In this work, we hypothesize that the predicted ionization efficiency could be used to estimate LoD and thereby enable evaluating the suitability of the LC/ESI/HRMS nontargeted method for the detection of suspected chemicals even if analytical standards are lacking. For this, 221 representative compounds were selected from the NORMAN SusDat list (S0), and LoD values were determined by using 4 complementary approaches. The LoD values were correlated to ionization efficiency values predicted with previously trained random forest regression. A robust regression was then used to estimate LoD values of unknown features detected in the nontargeted screening of wastewater samples. These estimated LoD values were used for prioritization of the unknown features. Furthermore, we present LoD values for the NORMAN SusDat list with a reversed-phase C18 LC method.
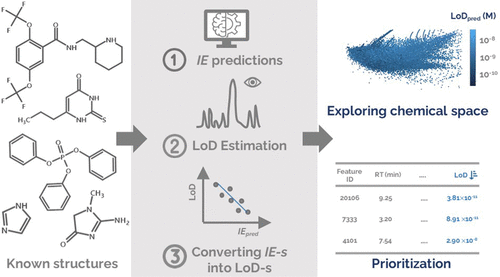
Gas Phase Reactivity of Isomeric Hydroxylated Polychlorinated Biphenyls
Emma H Palm, Josefin Engelhardt, Sofja Tshepelevitsh, Jana Weiss, Anneli Kruve
JASMS 2024
Identification of stereo- and positional isomers detected with high-resolution mass spectrometry (HRMS) is often challenging due to near-identical fragmentation spectra (MS2), similar retention times, and collision cross-section values (CCS). Here we address this challenge on the example of hydroxylated polychlorinated biphenyls (OH-PCBs) with the aim to (1) distinguish between isomers of OH-PCBs using two-dimensional ion mobility spectrometry (2D-IMS) and (2) investigate the structure of the fragments of OH-PCBs and their fragmentation mechanisms by ion mobility spectrometry coupled to high-resolution mass spectrometry (IMS-HRMS). The MS2 spectra as well as CCS values of the deprotonated molecule and fragment ions were measured for 18 OH-PCBs using flow injections coupled to a cyclic IMS-HRMS. The MS2 spectra as well as the CCS values of the parent and fragment ions were similar between parent compound isomers; however, ion mobility separation of the fragment ions is hinting at the formation of isomeric fragments. Different parent compound isomers also yielded different numbers of isomeric fragment mobilogram peaks giving new insights into the fragmentation of these compounds and indicating new possibilities for identification. For spectral interpretation, Gibbs free energies and CCS values for the fragment ions of 4′-OH-CB35, 4′-OH-CB79, 2-OH-CB77 and 4-OH-CB107 were calculated and enabled assignment of structures to the isomeric mobilogram peaks of [M-H-HCl]− fragments. Finally, further fragmentation of the isomeric fragments revealed different fragmentation pathways depending on the isomeric fragment ions.
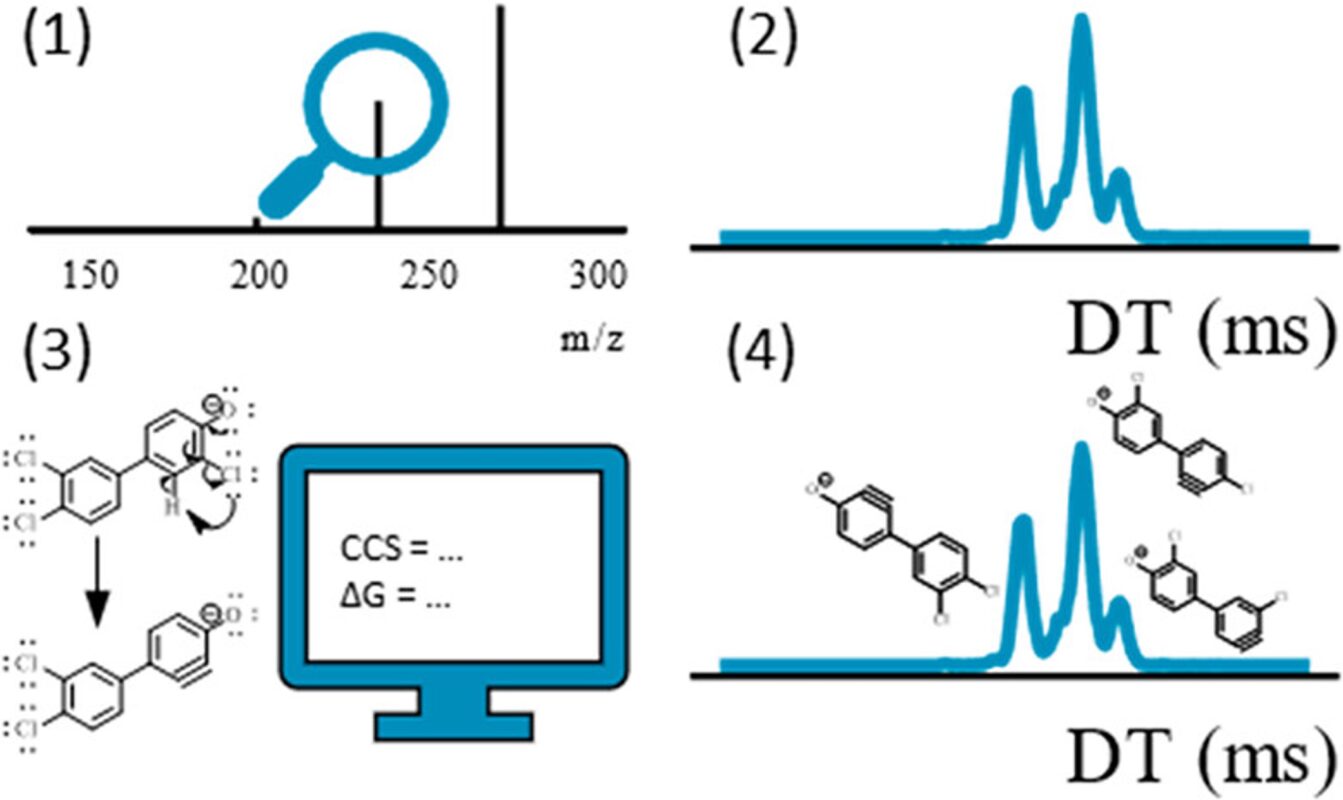
Predicting the Activity of Unidentified Chemicals in Complementary Bioassays from the HRMS Data to Pinpoint Potential Endocrine Disruptors
Ida Rahu, Meelis Kull, Anneli Kruve
J. Chem. Inf. Model 2024
The majority of chemicals detected via nontarget liquid chromatography high-resolution mass spectrometry (HRMS) in environmental samples remain unidentified, challenging the capability of existing machine learning models to pinpoint potential endocrine disruptors (EDs). Here, we predict the activity of unidentified chemicals across 12 bioassays related to EDs within the Tox21 10K dataset. Single- and multi-output models, utilizing various machine learning algorithms and molecular fingerprint features as an input, were trained for this purpose. To evaluate the models under near real-world conditions, Monte Carlo sampling was implemented for the first time. This technique enables the use of probabilistic fingerprint features derived from the experimental HRMS data with SIRIUS+CSI:FingerID as an input for models trained on true binary fingerprint features. Depending on the bioassay, the lowest false-positive rate at 90% recall ranged from 0.251 (sr.mmp, mitochondrial membrane potential) to 0.824 (nr.ar, androgen receptor), which is consistent with the trends observed in the models’ performances submitted for the Tox21 Data Challenge. These findings underscore the informativeness of fingerprint features that can be compiled from HRMS in predicting the endocrine-disrupting activity. Moreover, an in-depth SHapley Additive exPlanations analysis unveiled the models’ ability to pinpoint structural patterns linked to the modes of action of active chemicals. Despite the superior performance of the single-output models compared to that of the multi-output models, the latter’s potential cannot be disregarded for similar tasks in the field of in silico toxicology. This study presents a significant advancement in identifying potentially toxic chemicals within complex mixtures without unambiguous identification and effectively reducing the workload for postprocessing by up to 75% in nontarget HRMS.
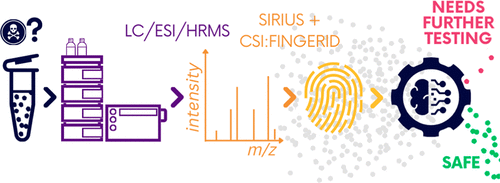
Online and Offline Prioritization of Chemicals of Interest in Suspect Screening and Non-targeted Screening with High-Resolution Mass Spectrometry
Drew Szabo, Travis M Falconer, Christine M Fisher, Ted Heise, Allison L Phillips, Gyorgy Vas, Antony J Williams, Anneli Kruve
Anal Chem 2024
DOI: 10.1021/acs.analchem.3c05705
Recent advances in high-resolution mass spectrometry (HRMS) have enabled the detection of thousands of chemicals from a single sample, while computational methods have improved the identification and quantification of these chemicals in the absence of reference standards typically required in targeted analysis. However, to determine the presence of chemicals of interest that may pose an overall impact on ecological and human health, prioritization strategies must be used to effectively and efficiently highlight chemicals for further investigation. Prioritization can be based on a chemical’s physicochemical properties, structure, exposure, and toxicity, in addition to its regulatory status. This Perspective aims to provide a framework for the strategies used for chemical prioritization that can be implemented to facilitate high-quality research and communication of results. These strategies are categorized as either “online” or “offline” prioritization techniques. Online prioritization techniques trigger the isolation and fragmentation of ions from the low-energy mass spectra in real time, with user-defined parameters. Offline prioritization techniques, in contrast, highlight chemicals of interest after the data has been acquired; detected features can be filtered and ranked based on the relative abundance or the predicted structure, toxicity, and concentration imputed from the tandem mass spectrum (MS2). Here we provide an overview of these prioritization techniques and how they have been successfully implemented and reported in the literature to find chemicals of elevated risk to human and ecological environments. A complete list of software and tools is available from https://nontargetedanalysis.org/.
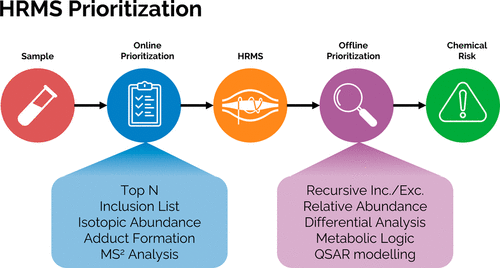
Closing the Organofluorine Mass Balance in Marine Mammals Using Suspect Screening and Machine Learning-Based Quantification
Mélanie Z. Lauria, Helen Sepman, Thomas Ledbetter, Merle Plassmann, Anna M. Roos, Malene Simon, Jonathan P. Benskin, Anneli Kruve
ES&T 2024
High-resolution mass spectrometry (HRMS)-based suspect and nontarget screening has identified a growing number of novel per- and polyfluoroalkyl substances (PFASs) in the environment. However, without analytical standards, the fraction of overall PFAS exposure accounted for by these suspects remains ambiguous. Fortunately, recent developments in ionization efficiency (IE) prediction using machine learning offer the possibility to quantify suspects lacking analytical standards. In the present work, a gradient boosted tree-based model for predicting log IE in negative mode was trained and then validated using 33 PFAS standards. The root-mean-square errors were 0.79 (for the entire test set) and 0.29 (for the 7 PFASs in the test set) log IE units. Thereafter, the model was applied to samples of liver from pilot whales (n = 5; East Greenland) and white beaked dolphins (n = 5, West Greenland; n = 3, Sweden) which contained a significant fraction (up to 70%) of unidentified organofluorine and 35 unquantified suspect PFASs (confidence level 2–4). IE-based quantification reduced the fraction of unidentified extractable organofluorine to 0–27%, demonstrating the utility of the method for closing the fluorine mass balance in the absence of analytical standards.
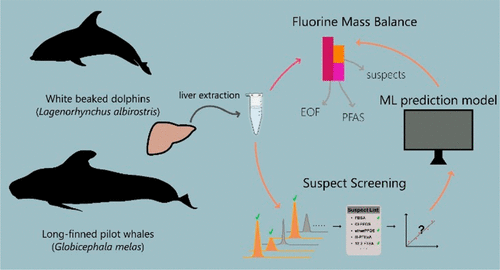